Multi-scale mechanistic modeling to improve outcomes in cancer immunotherapy
Faculty: Mohit Kumar Jolly (BSSE), Sudha Kumari (Microbiology & Cell Biology)
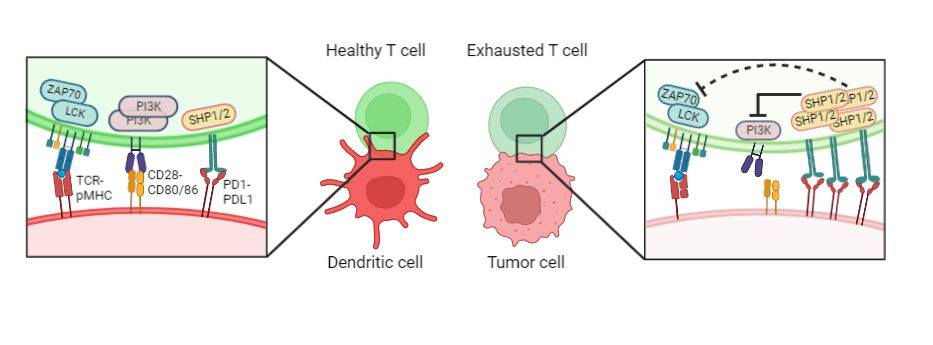
T cells are a subset of immune cells that patrol the body in search of rogue cells to ensure that the immune system is functioning properly. Over the last few years, immunotherapy has been gaining traction to treat cancer by improving T cell responses using monoclonal antibodies against PD1 or it’s binding partner anti-PDL1, thereby prolonging patient survival. Despite the massive success of immunotherapy, many patients fail to respond to such immunotherapy.
The reasons for immunotherapy failure are many. First, during cancer, effector T cells can become exhausted or dysfunctional. T-cell exhaustion is mainly characterized by their increased expression of immune checkpoints like PD1 and CTLA4, reduced cytokines, decreased proliferation capacity and cytotoxicity, and eventually clonal deletion of the same. Second, signalling pathways governing the levels and action of immune checkpoint markers is convoluted and involves various intertwined feedback loops, thus making the emergent dynamics of underlying crosstalk non-intuitive.
A systems-level approach – including mechanistic mathematical modeling and experimental validation – is therefore necessary to understand the fine balance between the expression levels of diverse receptors and their binding partners that allows success in immunotherapy. Such models should incorporate multi-scale interactions, i.e. cell-cell crosstalk between tumor and immune cells, and intracellular reprogramming of tumor cells and immune cells This project will deal with developing mathematical models of signaling pathways (receptor-ligand interactions) and downstream networks to develop a platform that can be used to better understand why specific immunotherapy have failed so far, and test treatment options in silico.
References: