Deep Learning for Seismic Imaging Toward the acceleration of geophysical inversion workflows
In earth sciences, determining subsurface properties from am- bient vibrations in the subsurface is commonly known as pas- sive imaging, which is a challenging task owing to the uncer- tainties in the seismic sources. However, as these experiments typically record an abundance of data, imaging algorithms that learn the underlying source-signal structure from the data via representation learning stand out from the rest. Most of the current passive imaging methods are physics-based in the sense that they aim to remove the source uncertainties us- ing the physics of the wave propagation, without the learning component. On the other hand, a straight forward appli- cation of representation learning algorithms from disciplines like computer vision suffer in sciences due to the lack of in- terpretability. In the view of the above, this PhD research will focus on the development of data-driven deep-learning (DL) solutions for seismic inversion including reflectivity and ve- locity model building. Specifically, hybrid methods that make use of physics-embedded neural networks for solving prob- lems pertaining to seismic imaging will be developed.
Fast and accurate solutions to large-scale seismic inverse prob- lems are critical for geophysical exploration and solid Earth geoscience tasks, which currently rely exclusively on model- based numerical solutions that require overwhelming com- putational resources, human-based intervention, and iterative workflows that often last months until convergence. The expo- nential growth in available seismic data, in conjunction with the outstanding success of DL in solving inverse problems, is turning DL into an effective tool for seismic inversion.
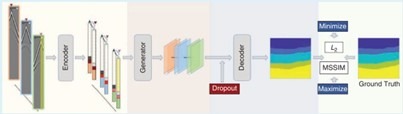
References
- Pawan Bharadwaj, Matt Li, and Laurent Demanet, (2020), “SymAE: An autoencoder with embedded physical symmetries for passive time-lapse monitoring,” SEG Technical Program Expanded Abstracts : 1586-1590.
- Fangshu Yang and Jianwei Ma, (2019), “Deep-learning inversion: A next-generation seismic velocity model building method,” GEOPHYSICS 84: R583-R599.
- S. Li, B. Liu, Y. Ren, Y. Chen, S. Yang, Y. Wang, and P. Jiang, “Deep-learning inversion of seismic data,” IEEE Trans. Geosci. Remote Sens., vol. 58, no. 3, pp. 1–15, 2020.
This position provides a unique opportunity for students to work in areas spanning computational seismic imaging, inverse problems and scientific deep-learning methods. Our research eRorts will lead to the development of novel signal-processing algorithms with application to seismology, medical imaging, and other scientific domains.
Desired qualifications
Applicants with strong background in applied mathemat- ics, physics, geological sciences, computer science, and engineering are invited to apply. It is expected that the student will also strengthen the existing collaborations of the supervisors with the energy industry and other stake- holders. The student will have the opportunity to learn and discuss with signal-processing experts and seismol- ogists from the institute.